Nvidia’s upcoming GPU, expected around 2026–27, will feature 88 custom Arm cores connected by NVLink-C2C at 8 TB/s and 13TB of HBM4 memory, creating a tightly integrated architecture. Named Rubin, it will support autonomous robots and medical applications, while raising ethical concerns over potential misuse. Nvidia also plans a future Feynman chip. The company is advancing electro-optical links for faster data transfer and scaling down supercomputers with the DGX Spark system. Its robotics focus includes partnerships with DeepMind and Disney, signaling a strategic move into AI-driven robotics and general-purpose autonomous systems.
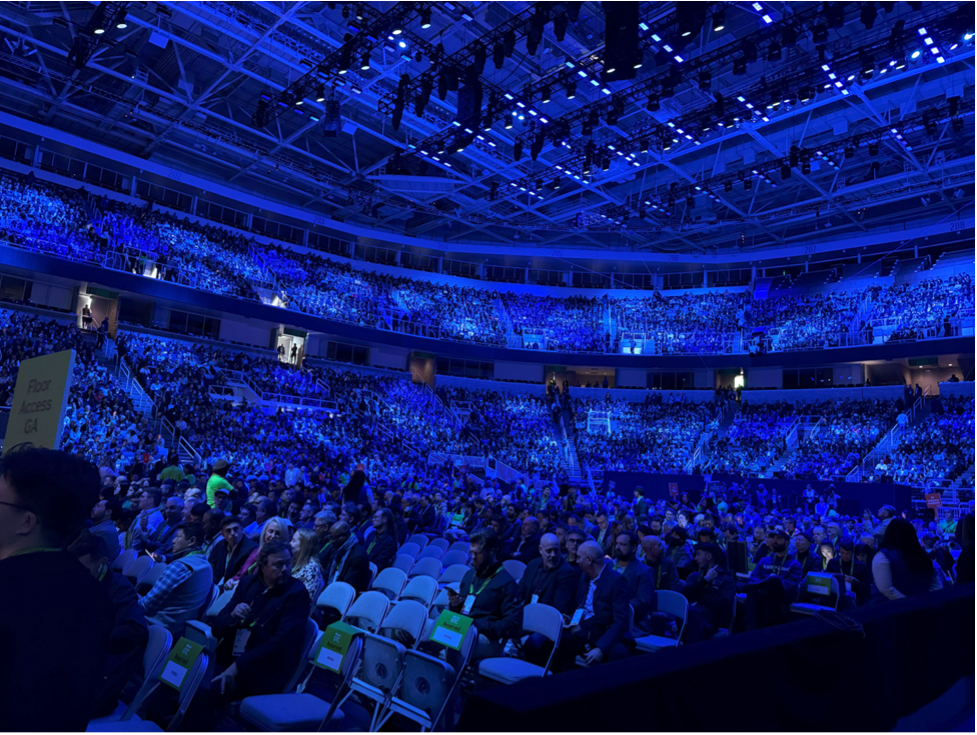
Nvidia’s next GPU, which could show up in 2026–27 if Nvidia can maintain its yearly cadence, will have 88 custom Arm cores running at 8 TB/s through NVLink-C2C to each other. That’s so fast that one core won’t really know or care where the other core is, it will think it is its own.
It will also have a massive 13TB of HBM4 so tightly coupled it will almost feel like cache to the little Arm RISC processors. This is a lot of processor and lot of memory so integrated it’s almost a processor in memory architecture—almost.
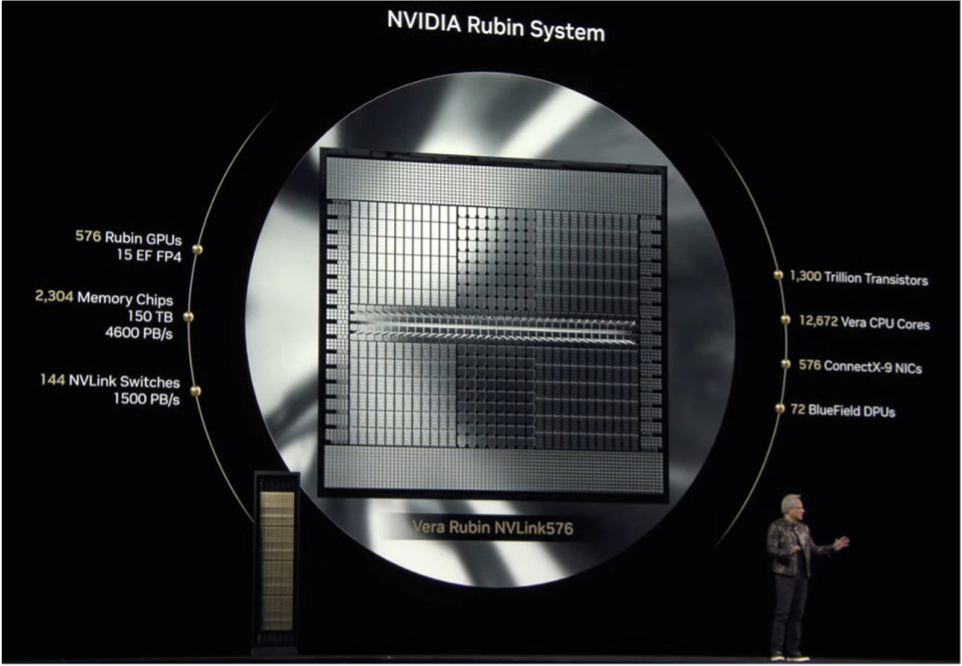
These are numbers that supercomputer designers of a decade ago couldn’t even imagine, and now they can have them, and lots of them, in water-cooled racks with optical cables connecting them. Will this enormous processing power be used for good or evil? Both, sadly. Medical research will make new leaps, and so will extraordinary lethal weapons that could end life on Earth in a few seconds. Will we have the wisdom and impulse control to manage them?
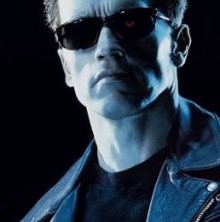
Rubin will also power autonomous robots that will change our lives forever. We may not get flying cars (and who really wants them?), but we will get helpers who can tend to the ill and aged, unburden the soccer mom, feed and walk the dog, and cut the grass. Teenagers will be made obsolete and ultimately eliminated. They will also build… other robots—didn’t Arnold Schwarzenegger make a few movies about this?
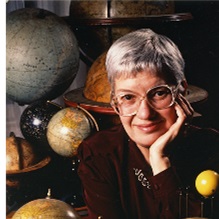
All of this, or most it, have nothing to with award-winning Vera Florence Cooper Rubin, an American astronomer who pioneered work on galaxy rotation rates. She uncovered the discrepancy between the predicted and observed angular motion of galaxies by studying galactic rotation curves and opened the window to dark matter. Had she had one of these processors, she probably would have done it 10 years sooner. Nvidia chose to honor her, as it has done with other scientific greats, in naming this new supercomputer chip after her.
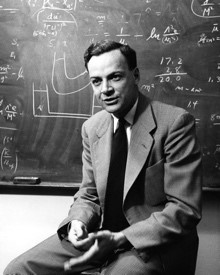
Another scientific giant was the physicist and mathematician Richard Feynman, who is known for his work in quantum mechanics, quantum electrodynamics, and particle physics in the 1950s, and later for his charming ability to explain incredibly complex concepts to freshman students.
The next GPU after the next GPU, expected in 2028, will be named Feynman, and although its specifications are logically vague at the time, if Nvidia follows its astonishing past few generations, we will be cautious about writing about it for fear of being accused of writing science fiction.
This is what Nvidia refers to as scaling up—making faster, bigger, processors. But those processors need a home and environment to present their power and worth.
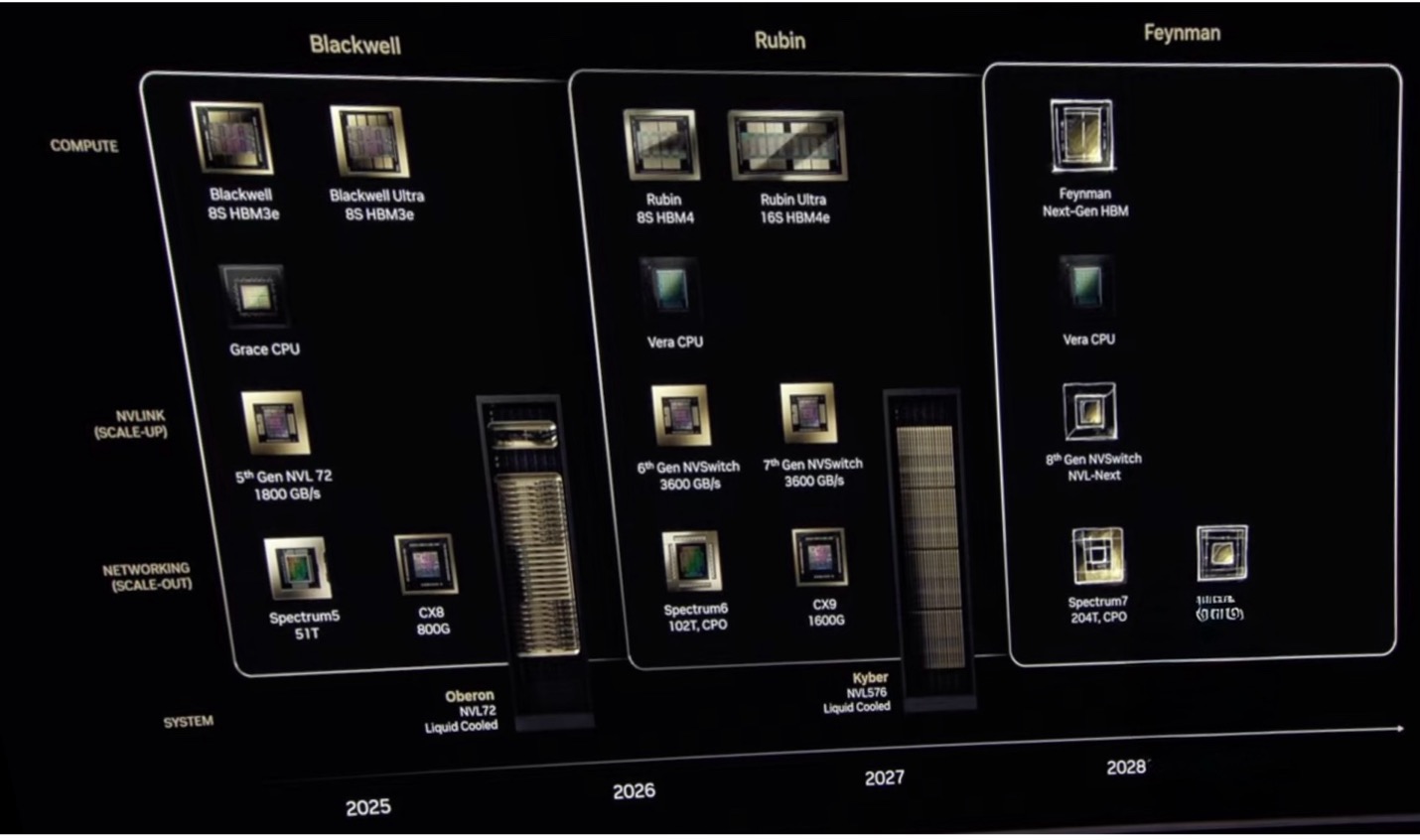
That involves a lot of mechanical design, chassis, racks, power distribution, and cooling. And, working with its long-term partner Foxconn, Nvidia has met that challenge, which Nvidia refers to as scaling up.
Part of the scaling up process includes things that are not specifically GPU-centric, like storage and communications.
At GTC during the Jensenathon, the pigs in a blanket server Huang announced that Nvidia was working with storage companies to incorporate GPUs in storage devices to make them smarter and faster. Nvidia introduced the AI Data Platform, a customizable reference design that storage developers are adopting to create what the company describes as a new type of infrastructure designed for AI inference workloads.
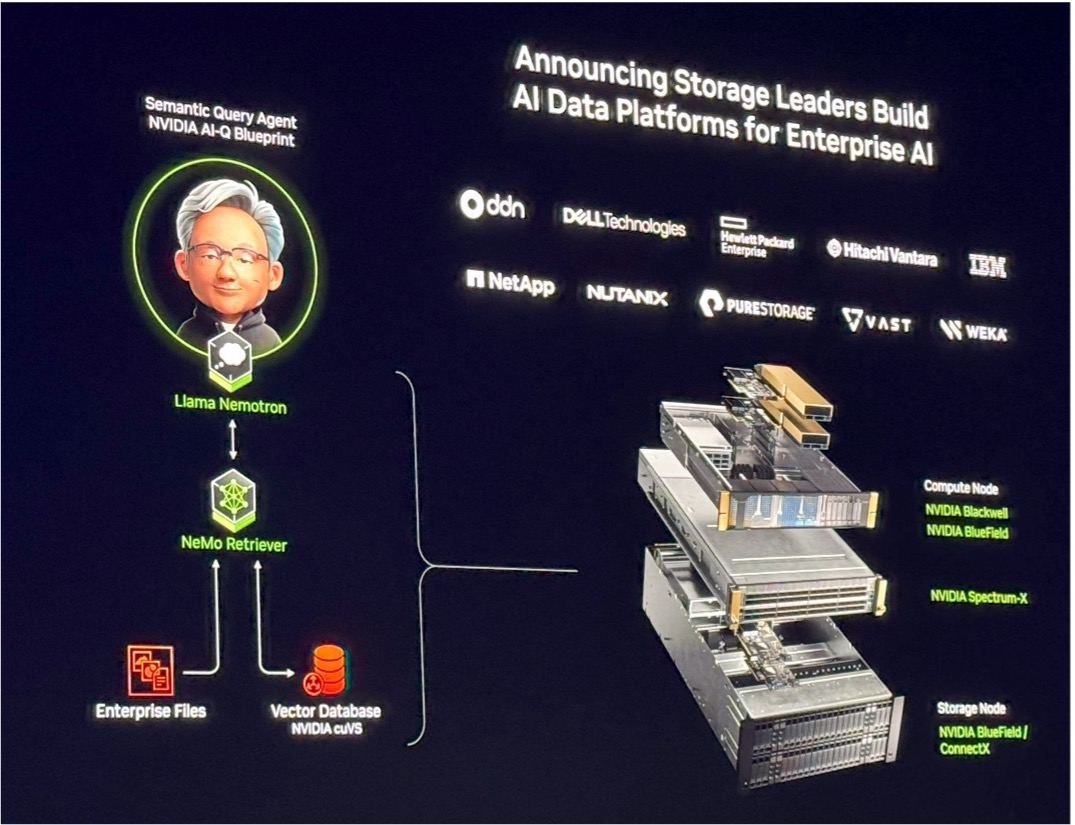
Not much more was said about that, but database companies have been using GPUs for superfast indexing for several years, and a specially adapted NVMe-to-GPU could be just the thing storage device suppliers were looking for to get the data in and out faster.
Not much more was said about that, but database companies have been using GPUs for superfast indexing for several years, and a specially adapted NVMe-to-GPU could be just the thing storage device suppliers were looking for to get the data in and out faster.
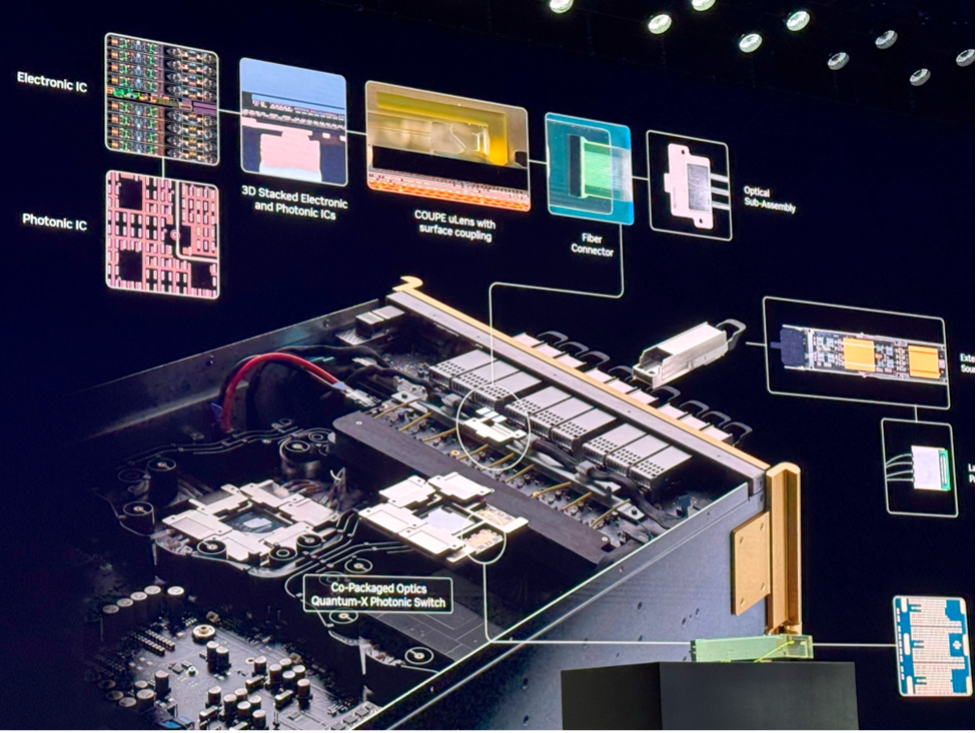
And living up to Huang’s credo of “Do the hard stuff,” Nvidia has taken on that challenge and announced a new, power-efficient electro-optical coupling system that can literally connect a GPU in a rack at the northeast end of a data center to a GPU in the southwest corner at 288.7 million meters per second—going through two or more transitions from GPU to chassis, to chassis to GPU. This is probably one of the most revolutionary things the company has done to date and put them so far out ahead of all the competition, it’s incredible.
Out, up, and down
In addition to scaling up and out, Nvidia also scaled down. It took the company’s first supercomputer chassis, the DGX-1, a big rack-mounted device and showed a 2025 version of the same device that would easily fit in a backpack and have a lot of room left over.
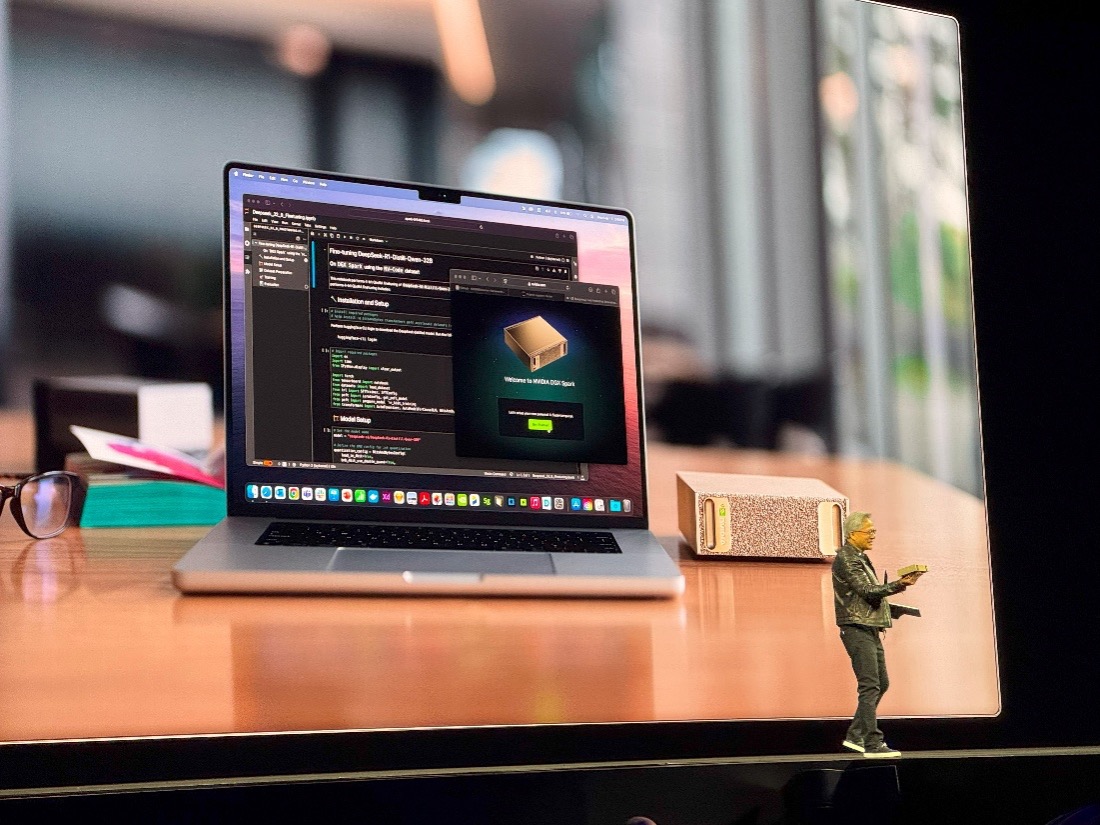
They call it DGX Spark (formally Project Digits); it’s powered by the Nvidia’s GB10 Grace Blackwell Superchip and delivers 1,000 AI TOPS of AI performance. Now, if an AI developer, maybe working on a company RAG, needs some local training power, she or he can jack into the DGX and get it, even if that person is in another country.
What will they do next?
Nvidia has been offering robot pathing design with its Omniverse for a few years now and regularly adding to the software stack for digital twins, which become a simulation of how a robot or many robots could or should behave in a factory, warehouse, or home, or a larger arena like a battlefield. As should be no surprise, the robot builders are incorporating GPUs into their device so they can do in situ learning in real time. And Nvidia is putting its resources behind such systems. The robot’s role extends beyond industrial, medical, scientific, and the home to entertainment as well, and Nvidia announced a partnership with DeepMind and Disney on AI Robotics Project and announced “the age of generalist robotics is here.”
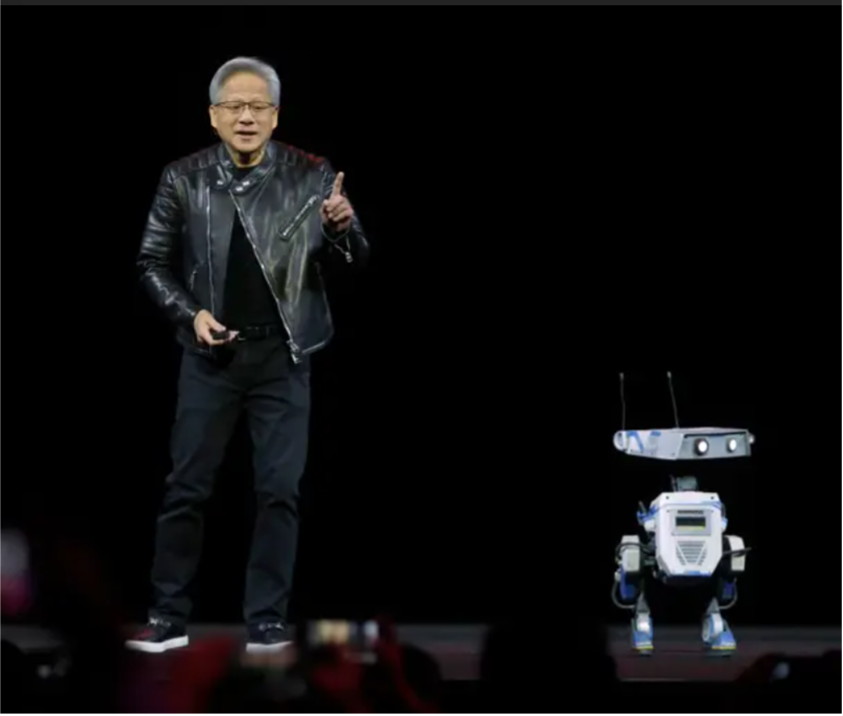
Blue’s Newton physics engine runs on two Nvidia computers and is built using Nvidia’s Warp framework. It is designed for robot learning and will support simulation environments like Google DeepMind’s MuJoCo and Nvidia Isaac Lab. The companies also plan to integrate Disney’s physics engine for additional capabilities.
But aside from the entertainment value, what Huang was clearly signaling was that Nvidia intends to be a major player in the potentially explosive robot industry, and if AI was Nvidia 2.0, robotics—from cars to medical assistants and space voyagers—will be Nvidia 3.0. Scoff, and you lose.
Wrapping it all up, Huang showed a family portrait, clearly demonstrating that Nvidia is a major high-performance computing company that also makes gaming, workstation, and automotive GPUs
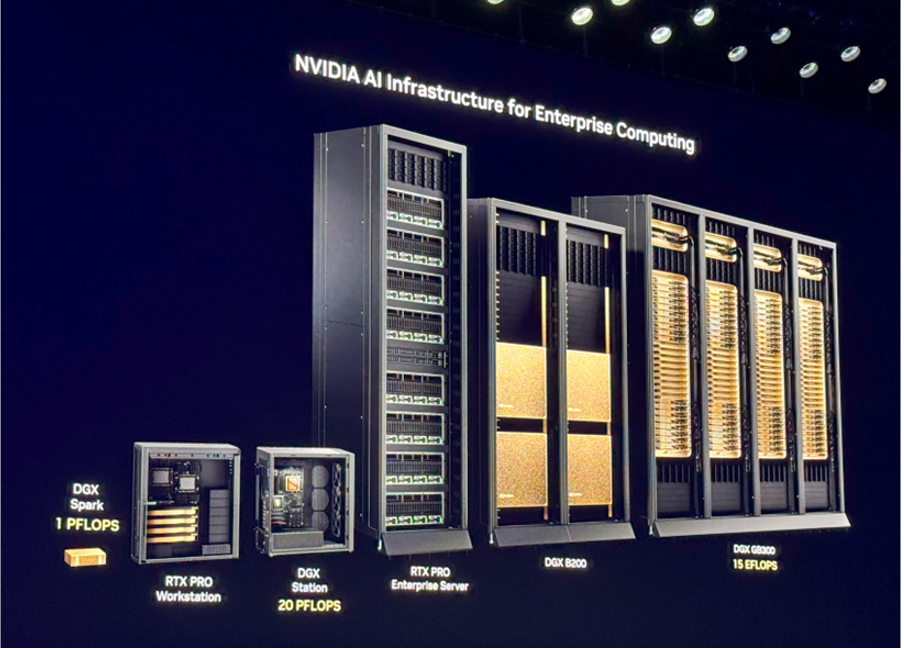
LIKE WHAT YOU’RE READING? INTRODUCE US TO YOUR FRIENDS AND COLLEAGUES.