Qualcomm demonstrated Stable Diffusion, a model for generating images, on a smartphone. The AI Model Efficiency Toolkit’s post-training quantization and Qualcomm AI Engine’s compilation were utilized for model compression and execution. This enabled the ability to run Stable Diffusion on smartphones in under 15 seconds, comparable to cloud latency. Furthermore, the research and optimizations will be integrated into the Qualcomm AI Stack, allowing scalability across different devices and models, making edge AI processing crucial for user privacy and cost-effective deployment.
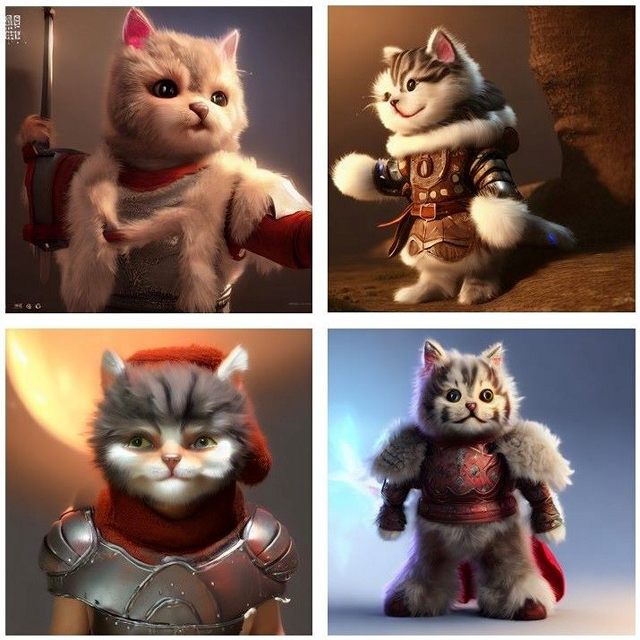
In Qualcomm’s blog post on its AI achievements, the company discussed demonstrating proof of concepts on commercial devices. They employed many aspects of AI, including applications, neural network models, algorithms, software, and hardware, and, says the company, fostered collaboration across disciplines within the organization.
To illustrate this, Qualcomm showcased Stable Diffusion. The researchers used the FP32 version 1–5 open-source model from Hugging Face and implemented optimizations through quantization, compilation, and hardware acceleration to make it compatible with the Snapdragon 8 Gen 2 mobile platform, used by smartphones. To reduce the model’s size from FP32 to INT8, the company used the AI Model Efficiency Toolkit’s (AIMET’s) post-training quantization, a tool developed by Qualcomm AI Research and now integrated into the recently announced Qualcomm AI Studio.
Quantization not only improves performance, but also saves power by enabling efficient execution on dedicated AI hardware and reducing memory bandwidth consumption. Quantization techniques, such as Adaptive Rounding (AdaRound), ensured that model accuracy remained unaffected at the lower precision without requiring retraining, said Qualcomm. These techniques were applied to all component models within Stable Diffusion, including the transformer-based text encoder, VAE decoder, and UNet, enabling the model to fit within device constraints.
For compilation, they used Qualcomm’s AI Engine direct framework, which maps the neural network into a program optimized for the target hardware. That framework sequences operations to enhance performance and minimize memory usage based on the hardware architecture and memory hierarchy of the Qualcomm Hexagon processor. Collaborative efforts between AI optimization researchers and compiler engineering teams, says the company, resulted in significant improvements in memory management for AI inference, leading to reduced run-time latency and power consumption. These optimizations have been integrated into the Qualcomm AI Engine, delivering continued benefits in the context of Stable Diffusion.
The Qualcomm AI Engine, in conjunction with the Hexagon processor in the Snapdragon 8 Gen 2, combined with microtile inferencing, enabled efficient execution of large models like Stable Diffusion. Further enhancements, such as those made for transformer models like MobileBERT, accelerated inference, particularly as multihead attention is utilized throughout the component models of Stable Diffusion. Consequently, Stable Diffusion could generate a 512×512-pixel image in under 15 seconds for 20 inference steps on a smartphone, which is comparable to cloud latency. Moreover, user text input remained unconstrained.
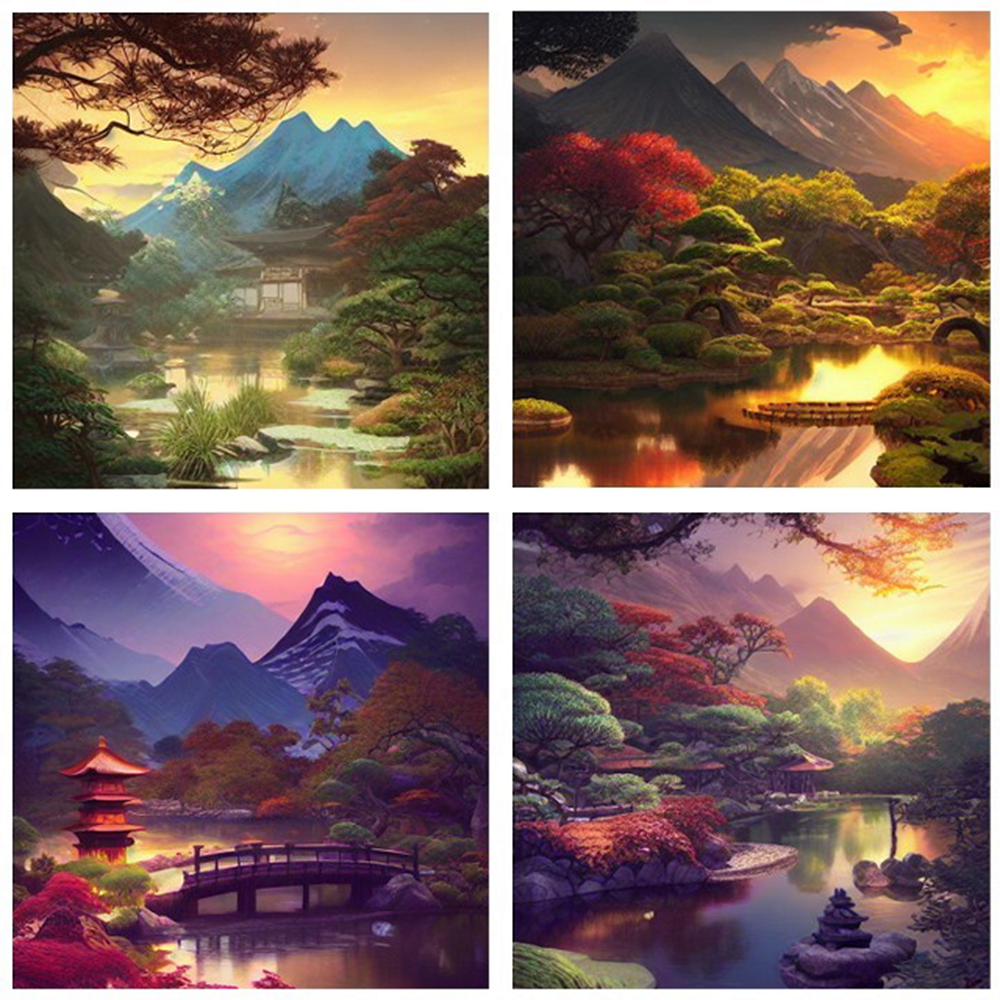
The connected intelligent edge, where large AI cloud models increasingly migrate towards running on edge devices, is rapidly becoming a reality. What was once considered impossible just a few years ago is now feasible. On-device processing with edge AI offers numerous benefits, including reliability, low latency, enhanced privacy, efficient use of network bandwidth, and cost-effectiveness.
While the Stable Diffusion model may appear sizable, it encapsulates a vast amount of knowledge pertaining to speech and visuals, enabling the generation of virtually any conceivable image. Furthermore, as a foundational model, Stable Diffusion extends beyond image generation with text prompts. It finds applications in various domains, including image editing, in-painting, style transfer, super-resolution, and more, delivering tangible impact. The ability to run the model entirely on the device, without requiring an internet connection, unlocks endless possibilities.
The research and optimization efforts that enabled this achievement will now be integrated into the Qualcomm AI Stack. Therefore, the optimizations implemented for Stable Diffusion on phones can also be applied to other platforms such as laptops, XR headsets, and virtually any Qualcomm Technologies-powered device.
What do we think?
While executing all AI processing in the cloud can be prohibitively expensive, AI processing on edge devices becomes interesting and possible. Utilizing edge AI processing, a user’s privacy should be safeguarded during the operation of Stable Diffusion and other generative AI models, as the input text and generated image never leave the device. That aspect holds significant value for consumers and enterprise applications. Also, the AI stack optimizations could lead to a decrease in the time to market for subsequent foundational models intended for edge deployment. So now I have a reason to upgrade my phone—to make cute and silly pictures.